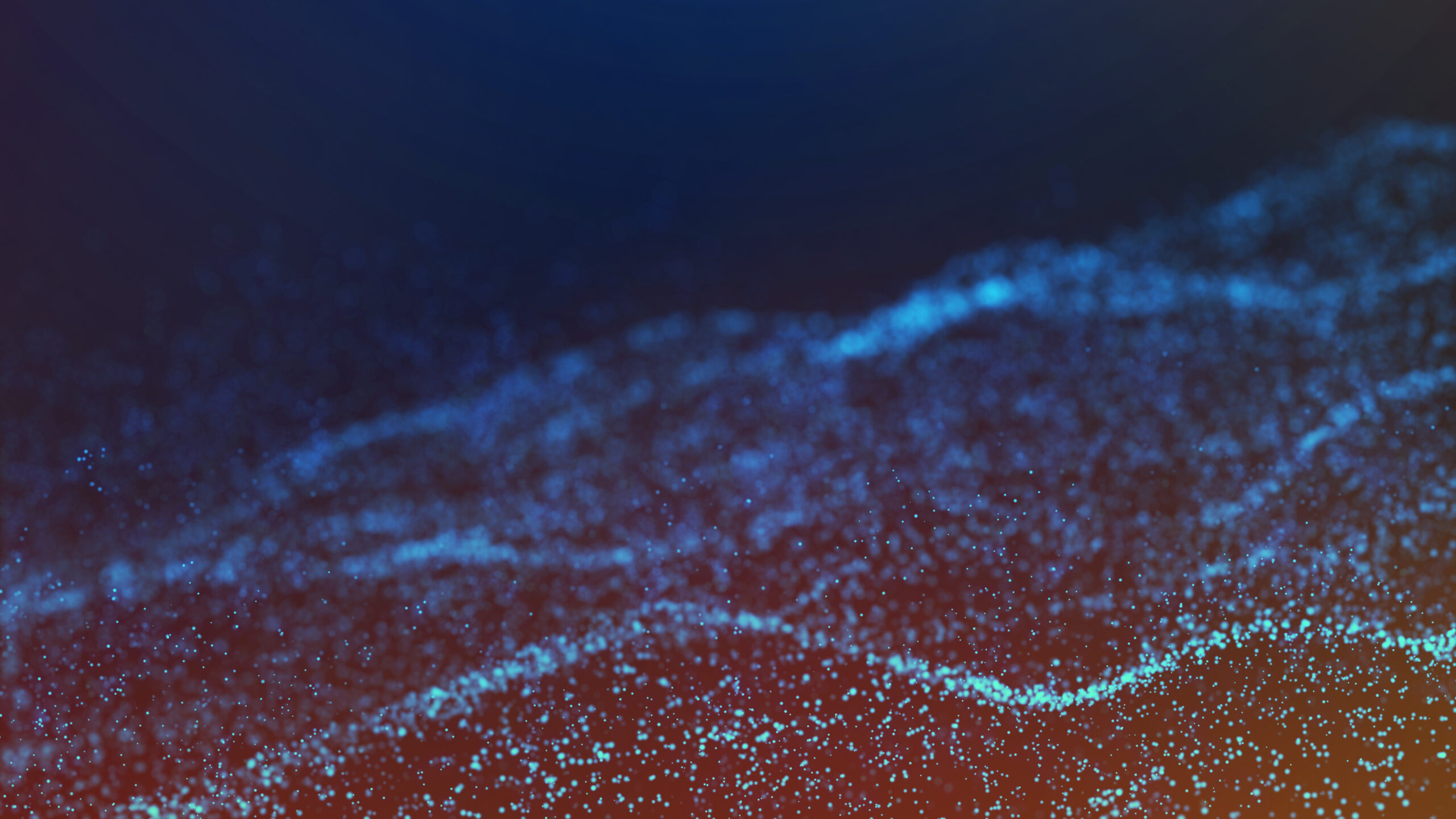
Data has become the primary fuel for companies. Innovation, transformation, differentiation, decarbonisation, operational excellence, profitability: everything is now based on data, and therefore on those who are able to make it speak, the data scientists. The demand for these profiles, which are already in short supply, will continue to grow and competition between startups, tech giants and large corporations will intensify to attract them.
Moreover, data scientists are like five-legged sheep, or rather three-legged sheep, experts in mathematical modelling, IT and the activities they work on. It will therefore be difficult to train a large number of them, especially when developers, architects and cybersecurity specialists are also needed.
In order to deepen the exploitation of data, it will therefore be necessary, on the one hand, to expand the teams by making data science more accessible. On the other hand, we will need to maximize the productivity of the few and expensive available specialists. AutoML is an answer to this double challenge.
What is AutoML?
AutoML aims to automate the tedious, repetitive and time-consuming tasks required to develop Machine Learning (ML) models. This work, which is now done by data scientists, consists of five successive steps that constitute the ML pipeline:
- Collect the data previously identified as relevant to the problem at hand.
- Prepare this data by purging it of outliers, correcting errors and gaps, and possibly enriching it with business knowledge to improve the model’s performance and robustness.
- Define the features (feature engineering), i.e., extract or construct from the data the parameters that the algorithm must consider.
- Develop and train the model based on an algorithm chosen for its suitability to the type of data and the nature of the problem.
- Test, optimize and validate the model, which must produce accurate and precise results. It must also provide sufficient guarantees in terms of explicability, reliability, robustness, fairness, usability, etc.
It is only after these five steps that the model can be deployed and made available to users. After that, it will be necessary to maintain it to take into account the evolution of the data, the quality of the results and the expectations of the business.
How does AutoML work?
To automate the ML pipeline, AutoML relies on reinforcement learning methods. Multiple pipelines are created to test various combinations of algorithms and features in parallel. At each iteration, the different models receive a learning score until the one that comes closest to the expected result emerges. The process stops after a predefined time or when certain relevance criteria are met.
What are the benefits of AutoML?
The first benefit of AutoML, the one that is most obviously targeted, is the saving of time (and therefore money). The automatic search for the best model saves data scientists laborious trial and error and allows them to obtain a result of acceptable quality much faster than they otherwise would. They can spend the time freed up developing more models or fine-tuning those with the greatest business impact. In terms of performance, we also note that AutoML algorithms tend to be more efficient than hand-coded models.
Automatically performing the ML pipeline steps also makes this modelling work accessible to an audience of business specialists who may not have all the necessary technical skills. AutoML can therefore accelerate and expand the adoption of Machine Learning with limited effort.
Finally, using a single, automated modelling method makes models more consistent and reliable, as they no longer depend on the practices or biases of individual data scientists. This is another important element in the perspective of generalizing Machine Learning at scale.
For which use cases is AutoML suitable?
AutoML allows industrializing the use of Machine Learning for classical use cases such as classification, regression, prediction and image recognition. In concrete terms, it can be used to anticipate behaviour (probability that a customer will leave the company, abandon a purchase, cancel a reservation, etc.), segment populations, detect fraud, predict the imminence of an event (predictive maintenance, etc.) or establish sales forecasts.
To find more about the potential uses of AutoML, please check out our article: “What is AutoML?“.
What are the limitations of AutoML?
AutoML greatly facilitates the work of data scientists, but it cannot totally replace their expertise in the choice of parameters that will enable them to go further in the optimization of the model. Moreover, it creates a “black box” effect since we don’t necessarily have all the elements that allow us to interpret the results of the model and how they were obtained. This can be an obstacle in certain contexts where this explicability is important, even required.
The evaluation of models is particularly problematic in the case of unsupervised learning. Finally, one must keep in mind that Machine Learning is not a panacea. It is only one approach to artificial intelligence among others. Data scientists have to determine if it is the most adapted to the nature of the problem, to the available data and to the expected level of accuracy, and it will always be up to them to bring their human guarantee to the results obtained.
Can AutoML replace data scientists?
Of course, AutoML can create any Machine Learning model on demand, but these models are not always flawless. Specialists must therefore intervene to verify that the model corresponds to the problem. Upstream of the process, the identification of outliers during data cleaning also requires an excellent knowledge of the business. And AutoML, as we have already mentioned, is not adapted to all types of problems.
For all these reasons, AutoML appears to be a formidable tool for rapid prototyping of new models and industrialization of the best-known use cases that can be entrusted to new types of users, but it will probably not replace data scientists. Data scientists still have a long way to go because their expertise in the most advanced business, data or algorithmic aspects is irreplaceable. On the other hand, thanks to AutoML, they can become even more efficient in their job.
How can I learn more?
This article is a part of a greater series centred around the technologies and themes found within the first edition of the Devoteam TechRadar. To read further into these topics, please download the TechRadar.
u003ch3u003eu003cstrongu003eWant to know more about AutoMl?u003c/strongu003eu003c/h3u003ernCheck out our TechRadar to see what our experts say about its viability in the market.
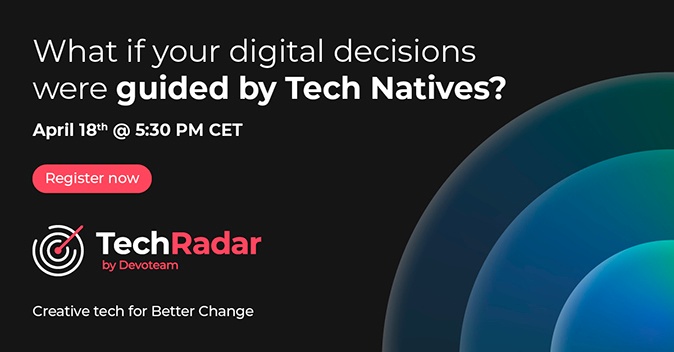